Application of adaptive local iterative filtering in gear fault identification
-
摘要:
针对齿轮实测信号因受噪声干扰而不能准确反映故障特征的问题,提出将自适应局部迭代滤波应用到齿轮故障识别中,与样本熵、灰色关联度相结合实现齿轮的故障识别。利用自适应局部迭代滤波将齿轮非平稳信号分解为有限个平稳的本质模态函数,通过计算各本质模态函数的样本熵,发现以齿轮系统的转频信号对应的本质模态函数的样本熵为界,前几个本质模态函数的样本熵能表征不同故障类型的特征;计算齿轮系统正常、齿面轻度磨损、齿面中度磨损和断齿4种工况下多个训练样本的样本熵的平均值,将其作为对应工况标准故障模式的参考值;计算待检测样本的样本熵与各状态下训练样本的样本熵平均值之间的灰色关联度,与待识别样本灰色关联度最大的标准故障模式即被认为是待识别样本的故障类型。实例分析结果表明,通过自适应迭代滤波能有效抑制模态混叠现象,发现明显的齿轮转频信号,而采用集合经验模式分解(EEMD)方法进行信号分解后,模态混叠现象比较明显,且在EEMD的分解结果中基本看不出齿轮的转频分量;4种工况的样本熵曲线形状存在明显差异,说明样本熵能有效表征齿轮故障特征的变化;灰色关联度方法能有效地将4种不同的故障类型进行分类识别,分类识别性能优于BP神经网络,对小样本数据具有较好的分类识别能力。
Abstract:In order to solve the problem that the measured signal of gears cannot accurately reflect the fault characteristics due to noise interference, adaptive local iterative filtering is proposed to be applied to gear fault identification, which is combined with sample entropy and grey correlation to realize gear fault identification. By using adaptive local iterative filtering to decompose the gear non-stationary signal into a finite number of stationary intrinsic mode functions, and calculating the sample entropy of each intrinsic mode function, it is found that the sample entropy of the first few intrinsic mode functions can represent different fault types, bounded by the sample entropy of the intrinsic mode function corresponding to the frequency conversion signal of the gear system. The average value of the sample entropy of multiple training samples under four working conditions of gear system, including normal, mild tooth surface wear, moderate tooth surface wear and broken tooth, is calculated and used as the reference value of the standard failure mode of the corresponding working condition. The grey correlation between the sample entropy of the sample to be detected and the average value of the sample entropy of the training samples under each condition is calculated, and the standard failure mode with the largest grey correlation with the sample to be identified is considered as the failure type of the sample to be identified. The results of the case analysis show that the adaptive iterative filtering can suppress the modal aliasing phenomenon effectively and find obvious gear frequency conversion signals. On the other hand, the modal aliasing phenomenon is more obvious after the signal decomposition by EEMD method, and the frequency conversion component of the gear is basically invisible in the decomposition results of EEMD method. The obvious differences in the shapes of the sample entropy curves of the four working conditions indicate that the sample entropy can represent the changes of gear fault characteristics effectively. The grey correlation method, which can classify and identify the four different fault types effectively, has better classification identification performance than that of BP neural network and has better classification identification ability for small sample data.
-
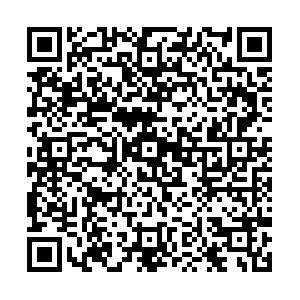
计量
- 文章访问数: 122
- HTML全文浏览量: 15
- PDF下载量: 8
- 被引次数: 0